
AJ Stuyvenberg

Jordan González
Building serverless applications offers developers flexibility, scalability, and a smooth development experience. But while serverless environments automatically scale with demand, they also introduce resource constraints that can impact performance and observability. At Datadog, we set out to overcome these challenges by reengineering our AWS Lambda extension to deliver high-fidelity telemetry with minimal overhead. In this post, we’ll explain how we reengineered our AWS Lambda extension—cutting cold starts by 82 percent, reducing memory usage by 40 percent, and shrinking the binary size from 55 MB to just 7 MB.
Datadog was a launch partner and has supported AWS Lambda Extensions since their launch in 2020. The Datadog Lambda Extension operates as a sidecar process that can collect logs, metrics, APM traces, profiles, and process information, then aggregate and relay that telemetry data asynchronously from the Lambda invocation lifecycle.
Initially, the Datadog Lambda Extension was built on top of our existing Datadog Agent, which is designed to collect resources for multiple hosts, pods, or even entire clusters. The Datadog Agent has to balance fairness between clients, juggle enormous throughput, and make use of caching and buffering techniques while aggregating data and flushing it back to Datadog.
These design choices limited our ability to minimize Datadog’s overhead—specifically, our impact on Lambda cold starts, CPU, and memory consumption—in the resource-constrained environment of Lambda.
The Datadog Serverless team spent weeks removing unused dependencies with build tags, using UPX to compress the binary, removing init methods where possible, and even exploring Go plugins to lazily load optional modules. While all of these changes helped in small ways, none proved sufficiently impactful as we found the performance floor to still be around 450-500 ms of additional cold start latency, which was unacceptable to us. It became clear that we had squeezed as much performance from a large, long-lived program as we could for the tiny, short-lived environment of Lambda.
When (and why) to rewrite software
My perspective on rewrites is that they’re hard. Infamously, hilariously hard.
Rewriting working software from scratch is fraught with risk and danger. Typically, engineers dramatically underestimate the size and scale of the effort. We often forget the subtle bugs—especially the implied system invariants that aren’t explicitly declared. These are embedded in design choices and can unexpectedly surface when a new path is chosen.
Rewrites often end up with a “second system syndrome” and a condition where both systems are supported, which is especially true in client-side software like libraries and, yes, Lambda extensions.
As the staff engineer for the Serverless group, my initial reaction to we should rewrite this is always to think no and inquire about other possible options. When I hear we should rewrite this in Rust—a language that our team had no prior experience with up to this point—my reaction was hard no. Yet there were some convincing arguments that led me to change my perspective.
Despite the risks, we realized that a rewrite—done right—could significantly improve performance.
Benefits of Rust for Lambda extensions
The AWS Lambda runtime is almost perfectly tailored for Rust, especially for Lambda extensions. Lambda extensions behave similarly to parent processes. If a registered Lambda extension crashes, it also takes down the corresponding Lambda function, triggering a sandbox reset and another painful cold start.
This means that Lambda extensions should never ever crash, so Rust’s memory safety features are an excellent safeguard preventing things like data race conditions between threads.
Of course, there are other benefits as well. Rust builds absolutely tiny binaries. A typical hello-world Go binary can be surprisingly large, as the standard library, garbage collector, glibc replacement, and runtime are quite expansive. Rust, on the other hand, produces tiny binaries, typically on the order of kilobytes or single-digit megabytes.
Additionally, in Lambda, the only target is Amazon Linux and x86/Arm architectures. This limits our system compatibility issues that can make Rust a more difficult choice for production applications.
Finally, Rust’s combination of excellent concurrency primitives and memory safety enforced at compile time allows developers, even those without extensive experience writing concurrent software, to build a reliable program. For the most part, if the program compiles, it’ll run without crashing.
After a short hackathon proof of concept, we decided to invest in our Lambda extension rewrite, which we called Project Bottlecap.
Project Bottlecap
The project codename Bottlecap was inspired by an excellent blog post called The Builder’s Guide to Better Mousetraps by Marc Brooker, an AWS VP/Distinguished Engineer. Brooker’s post is about recognizing the need to rewrite a certain piece of software, and how to create organizational buy-in to tackle the project.
According to his blog post, one important question to ask is, "Is my scale different? A $20 bottle capper, and a bottling plant do the same thing, at different scales."
We were solving a fundamentally different problem in Lambda than we were with our main Datadog Agent, it was a different scale, and we could take shortcuts that simplified the problem, such as not supporting syslog or balancing input from multiple clients.
Design constraints
We got to work starting with a small team building a prototype along specific design constraints that must be carefully balanced:
- We needed to minimize any impact on the running Lambda function handler code. The Lambda extensions API allows an extension to run after the function handler has returned its result to the waiting client. This minimizes the impact of telemetry collection on the user’s actual experience, which is important because the majority of Lambda functions are utilized as some kind of API endpoint.
- At the same time, we wanted to minimize any additional CPU time on top of the normal Lambda execution time, called post-runtime duration.
It’s far more difficult to remove bloat than to simply not introduce it in the first place, so we set up dashboards and monitors against our cold start overhead from the outset of the project. Every PR would be benchmarked, and any increases or discrepancies were addressed before merging. This led to us making some interesting tradeoffs—like manually writing AWS API calls and signatures instead of using available SDKs, which added too much of a performance penalty.
Finally, we needed to maximize optionality. Work in Lambda functions typically is bimodal—either small, fast API functions, or larger asynchronous batch processing systems. Therefore, we needed to support a variety of flush strategies, such as sending data periodically, or at the end of an invocation, or during an invocation:
- Flush at the end: This is ideal for infrequently called functions or tiny, CPU-intensive function workloads where you don’t want telemetry creation to steal limited CPU, but you do want pretty immediate telemetry data from every invocation.
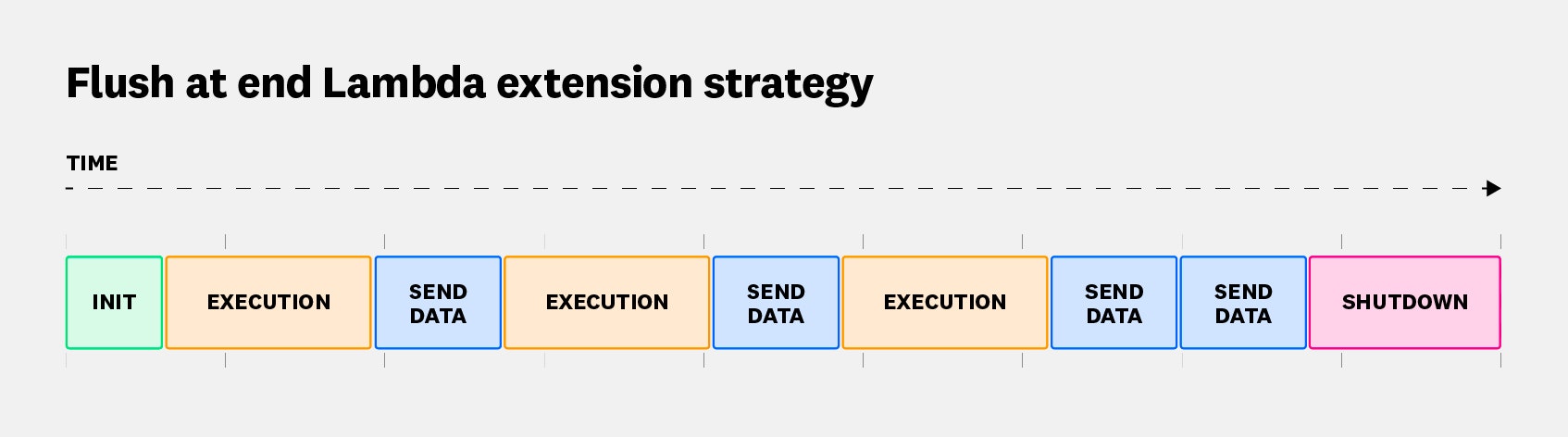
- Race the invocation: For long-running ETL jobs, encoding tasks, or large web crawling projects, it’s nice to have a live view of telemetry data coming in from the function, even if it hasn’t finished processing. The race strategy allows data to be sent at multiple points during the transaction, creating a live view of the ongoing function execution.
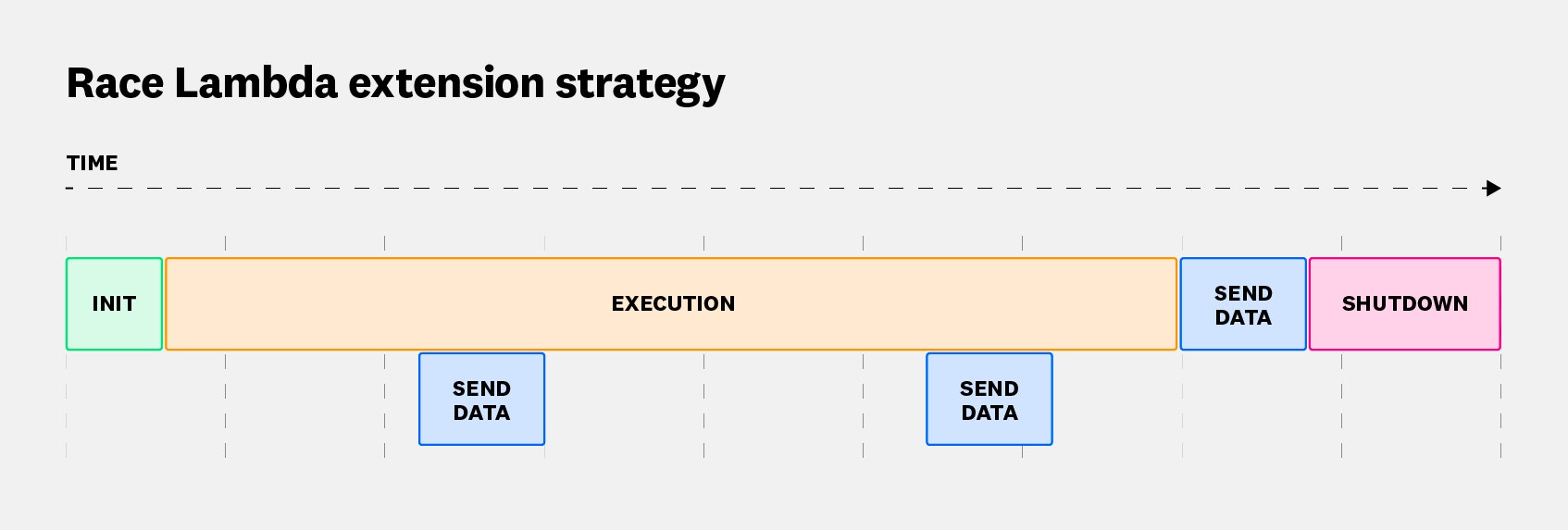
- Flush periodically: This is a great option to amortize the cost of flushing data across multiple invocations. With Datadog’s next-generation extension, the periodic time is fully adjustable so you can pick the right tradeoff between immediacy of telemetry data and minimal transfer costs. This also reduces CPU costs compared with flushing data at the end of every transaction.
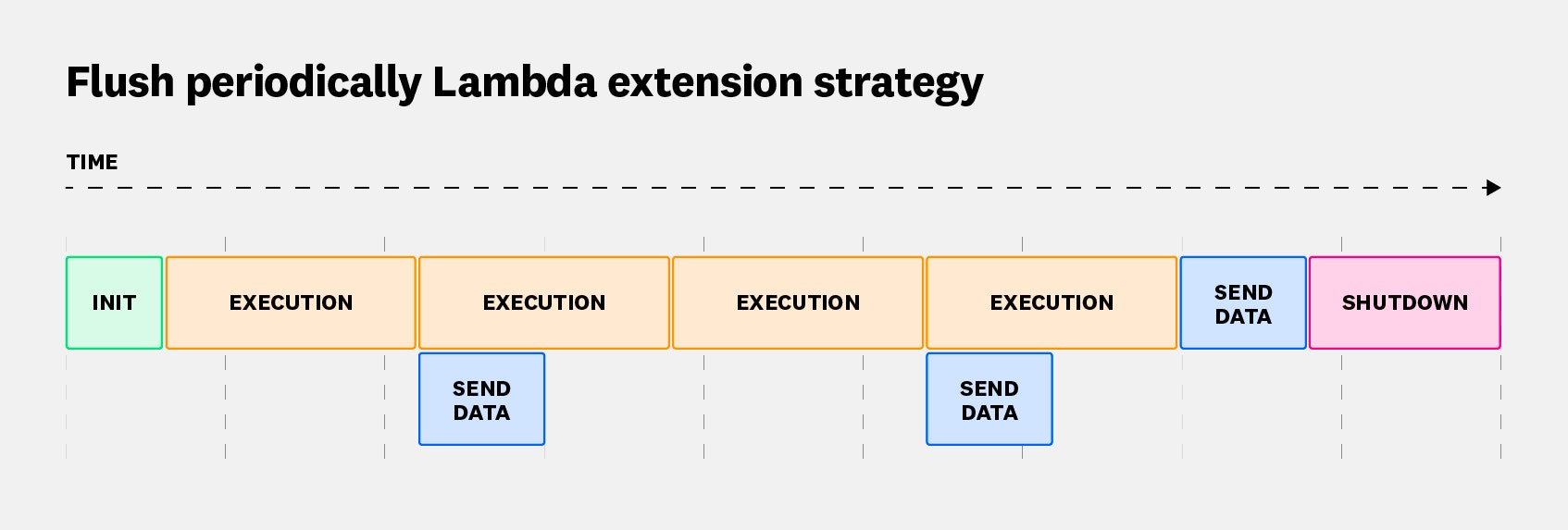
- Combination of strategies: Lambda function invocations aren’t all uniform! So Datadog’s next-generation extension actually combines these strategies. This way, if an unexpected long function execution occurs, you’ll receive telemetry information throughout the duration, while still moving to a periodic flush strategy after the long invocation completes.
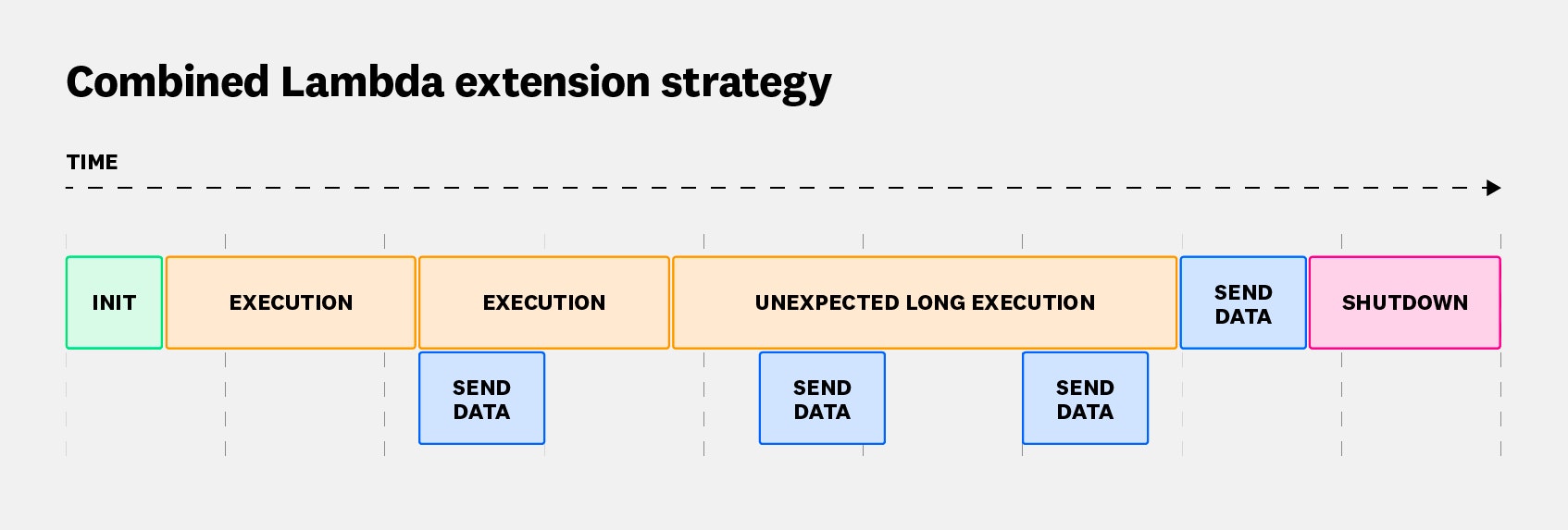
We’re continually rebalancing the flushing strategies and autodetection for optimal performance. Strategies are also configurable so users can pick what’s best for their workloads.
The results
We found our direct cold start impact dropped dramatically from approximately 450 ms to 70 ms when compared with our first-generation Lambda extension.
This meant that we realized an 82 percent cold start performance improvement with no compromises in functionality:
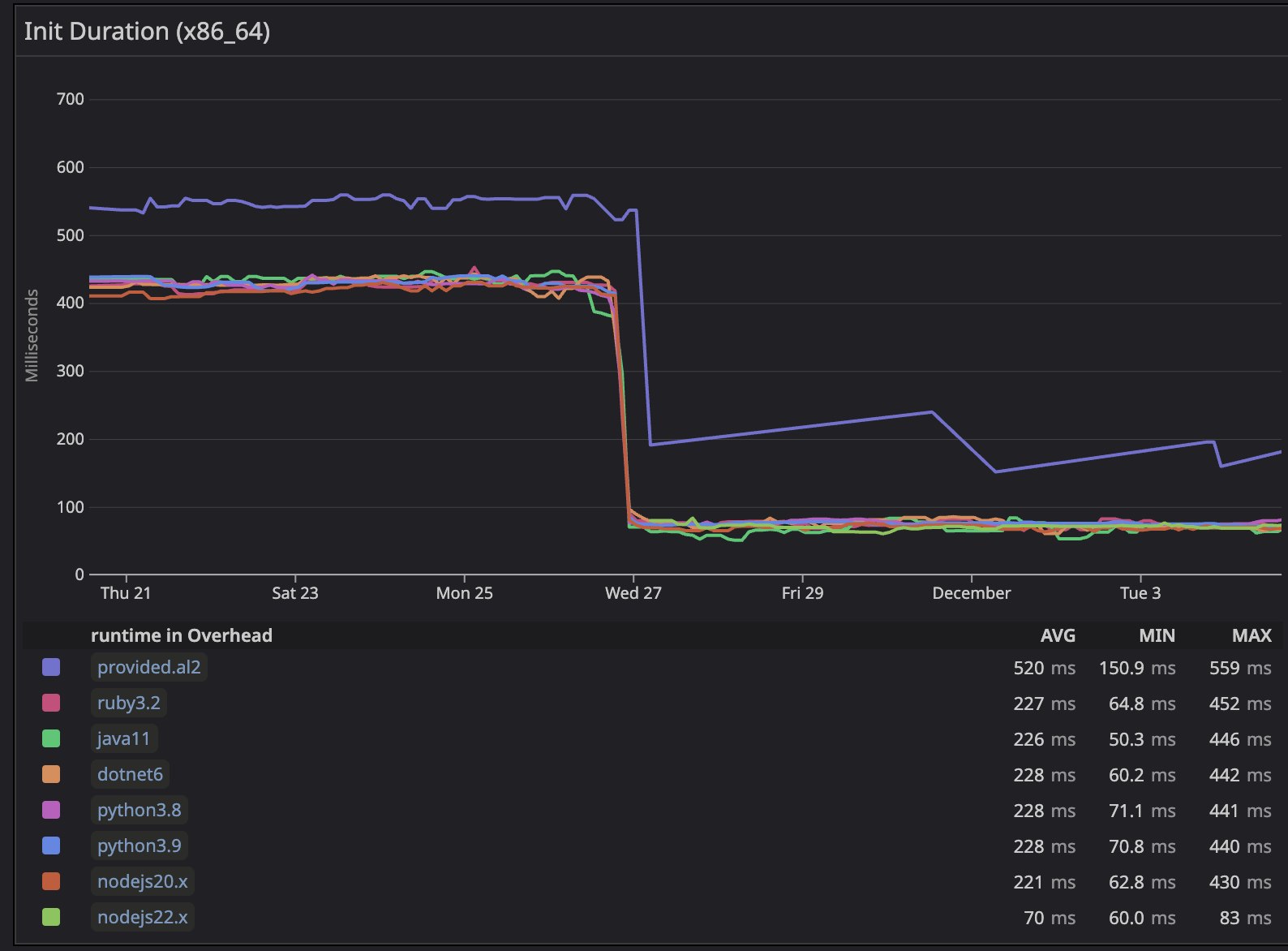
By designing specifically for Lambda and its miniscule available compute power alongside its specific lifecycle, we were able to reduce CPU consumption during the invoke phase and instead shift it afterward. This allowed our extension to run without interruptions and reduce overall billed duration and function response time.
We were also able reduce our memory impact by almost 40 percent, dropping from 128 MiB to 77 MiB:
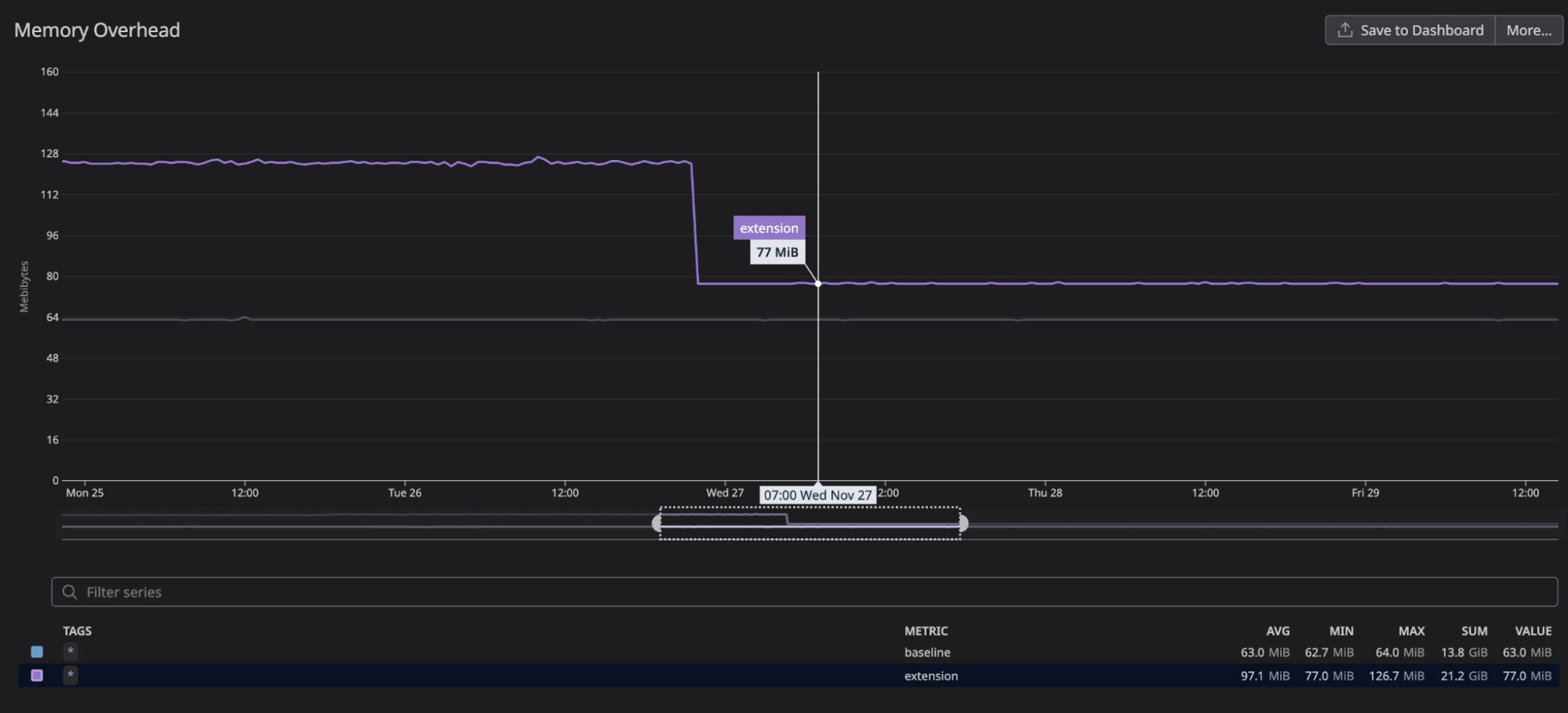
And we reduced our size impact from 55 MB down to 7 MB.
Shipping Bottlecap
Rolling out any new systems aimed to replace legacy ones is always challenging. We didn’t want to compromise the current behavior of any of our customers’ workloads. How could we seamlessly deliver the performance improvements to customers without affecting more exotic configurations that we weren’t ready to solve just yet?
We decided to approach this problem through a failover strategy, which would allow us to deliver customer value while having a gradual migration process. To accomplish this, keeping our legacy extension packaged along with our next-generation extension became imperative.
On every initialization, the next-generation Datadog Lambda Extension’s first task is detecting any incompatible configuration to decide whether it should fail-over into the legacy extension. This whole process spans approximately 4 ms, a worthwhile tradeoff that will go unnoticed most of the time.
This approach helped us understand which configurations were triggering fallbacks, so we added telemetry to track them. The telemetry gave us direct insight on which features our customers were using the most, leading us to prioritize support as we went. Unfortunately this also meant that we couldn't immediately realize the binary size gains by removing the legacy extension yet. But the benefits of supporting all users as we navigate this migration process outweigh the immediate benefits of a smaller total binary size.
Rust lessons learned
Overall, we feel that Rust was a natural fit for Lambda and that the goals of this project have been met or exceeded. We were pleased with the reduced binary size—from 55 MiB to 7 MiB—and extremely fast cold start times—dropping from approximately 450 ms to 70 ms—along with our ability to limit cases where the next-gen Datadog Lambda Extension can crash or fail.
Besides the syntax, moving from a Go project to Rust meant manually managing our own memory. Memory management was simpler than expected because Lambda extensions aggregate and flush data frequently. We tend to aggregate data over a short period of time and then flush it back to Datadog, releasing the memory as we go.
Rust’s memory acquisition and release mechanism is automated via RAII (Resource Acquisition Is Initialization). Memory is acquired when an object enters the scope, and freed when the object leaves. Because we don’t need to acquire a great deal of memory for the lifetime of the process, we didn’t have to carefully manage things like lifetimes or memory arenas with our project. We have targeted a case where we’d like to use a bump allocator and memory arena to improve p99 performance for narrow use cases, but we haven’t chosen to implement this yet.
New developers and recent graduates on our team managed to pick up Rust relatively easily, with the compiler helping guide them, which inspired confidence that the code they wrote would execute as intended.
What’s next
While we’re proud to deliver such a substantial improvement in cold start performance, pushing down long-tail p99 latency of Lambda requires more than simply faster cold starts. It requires analyzing the myriad of customer use cases and configurations, reducing memory allocations and lock contention, as well as working directly with the AWS Lambda team to improve Lambda extensions overall.
Since we began rolling out Bottlecap, we’ve continued to invest heavily on profiling and optimizing Datadog’s Lambda Extension to reduce CPU overhead, memory, and compute cost—a task made considerably easier thanks to Datadog’s monitoring and security platform.
If this type of work intrigues you, consider applying to work for our engineering team! We’re hiring!